Improvements of Stable Diffusion 3
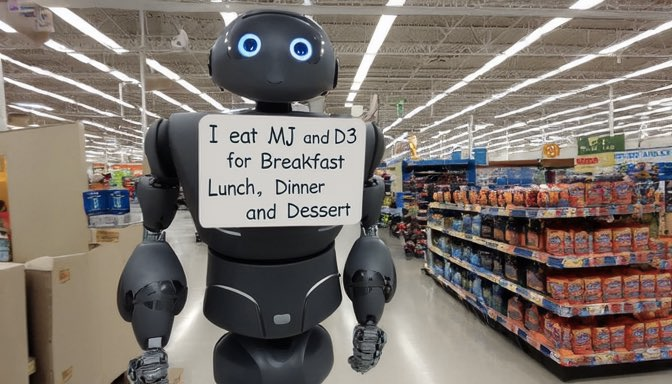
In the last article, we introduced the techniques behind Stable Diffusion 3. This text-to-image model created by Stability AI is the strongest so far. No matter if you want to generate fantastical multi-subject scenes or high-precision landscape photographs, absolutely nothing is off-limits.
Table of Contents
What improvements does Stable Diffusion 3 have?
Text Generation and Rendering
Stability AI emphasizes several key points of this version, with text rendering abilities being of paramount importance. They provide three images containing text, where the text is not only clear but error-free as well.

Generating the specified text based on a prompt has always been the prime headache of text-to-image models.
However, this time, the SD3 model well understood the prompt, correctly scrawling "go big or go home" upon the blackboard. Even further, the image is exceptionally realistic, with the positioning of far and near objects, lighting, and shadows appearing more than natural.
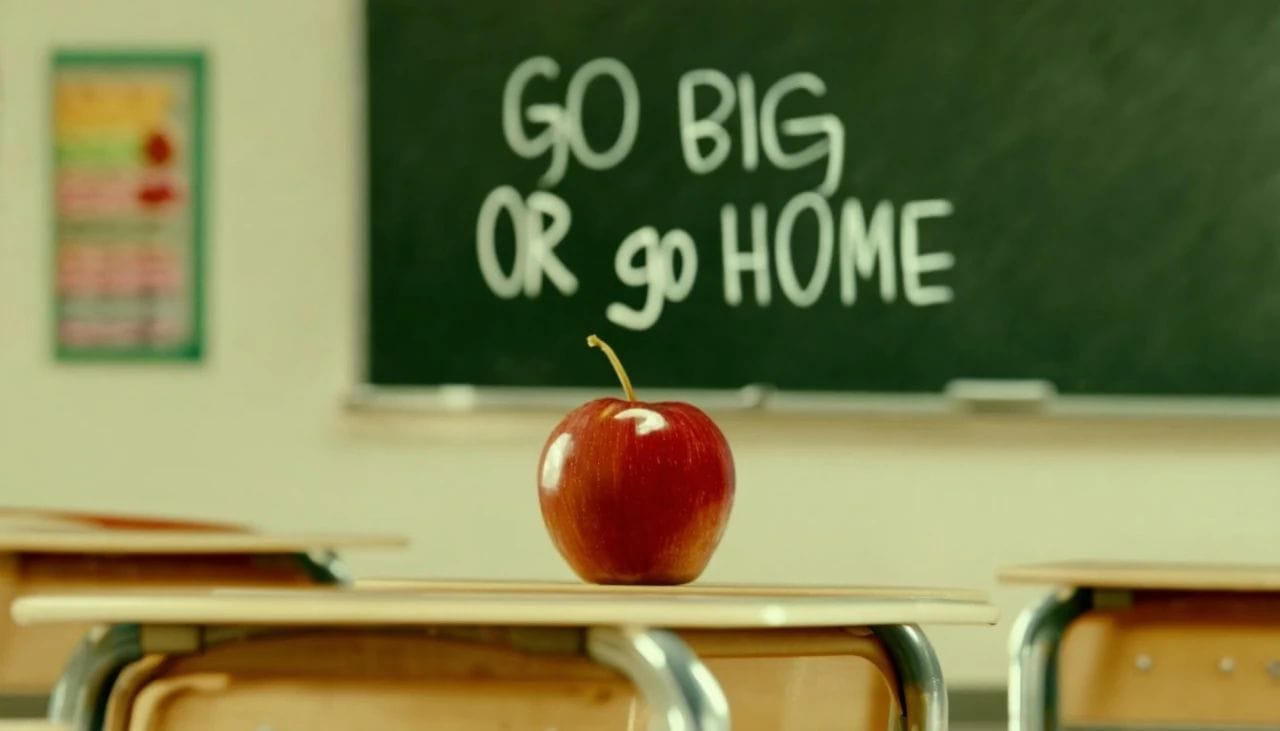
Multi-formatted text rendering, SD3 successfully met the challenge flawlessly. Irrespective of the CRT screen effect or the embossment effect on the fabric, though no clear prompts are provided in either, Stable Diffusion 3 demonstrates a masterful exhibition of the welcome and good night texts. Its integration with the images appears flawless.
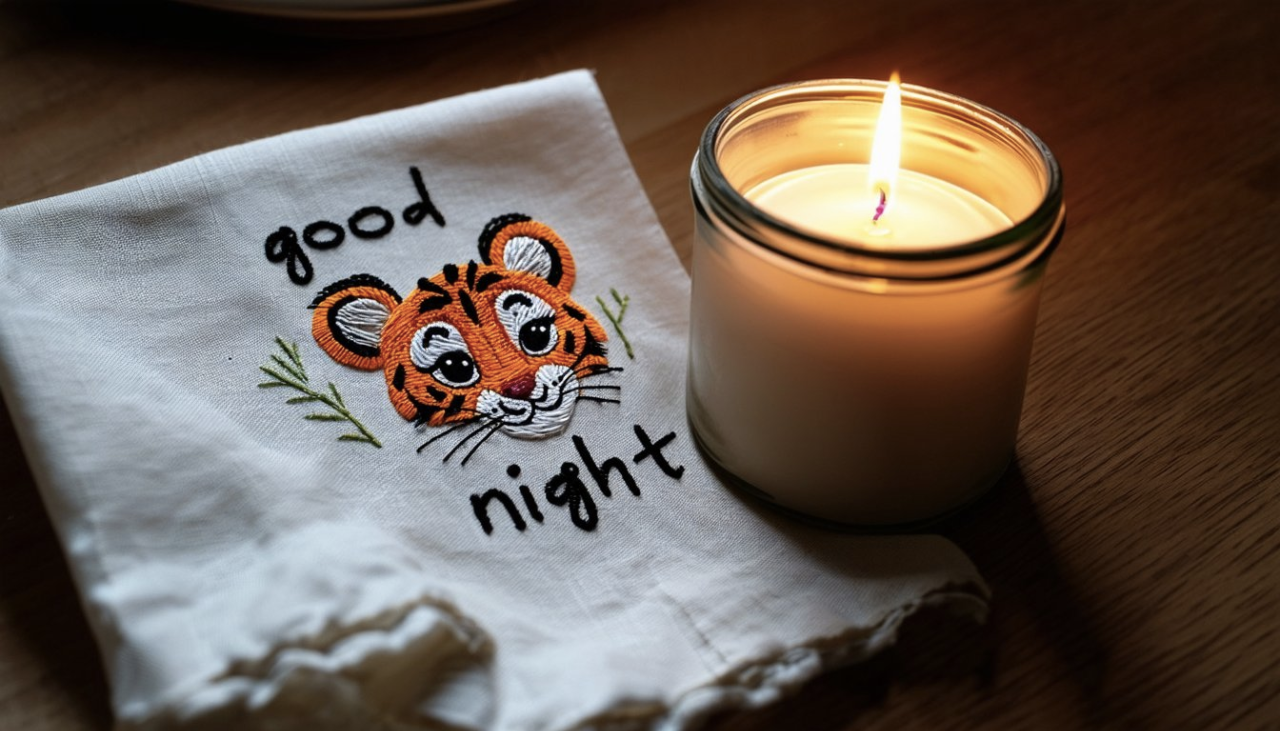
Prompt Following
The lack of prompt following in SDXL and Stable Cascade is a prominent issue. An innovative technique employed by DALLE 3 is its use of highly accurate image captions during training to effectively learn to follow prompts.
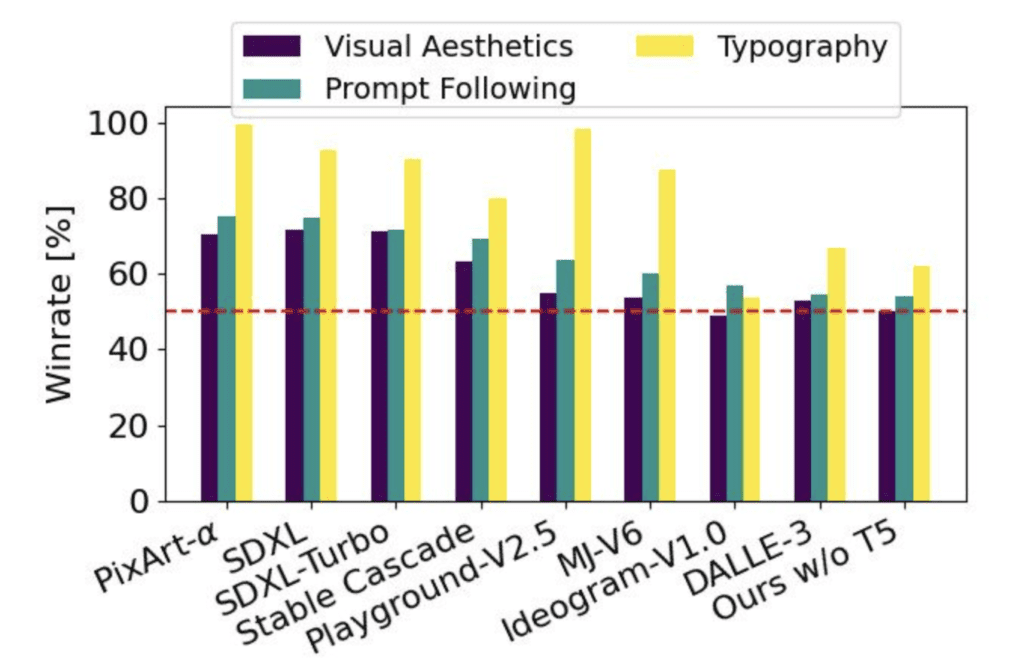
In Stable Diffusion 3, an additional highlight is "multi-subject generation": with a single sentence, SD3 can illustrate the multitude of worlds in the user's imagination. Stability AI provided several examples where SD3 successfully painted a picture upon a single prompt consisting of multiple elements:
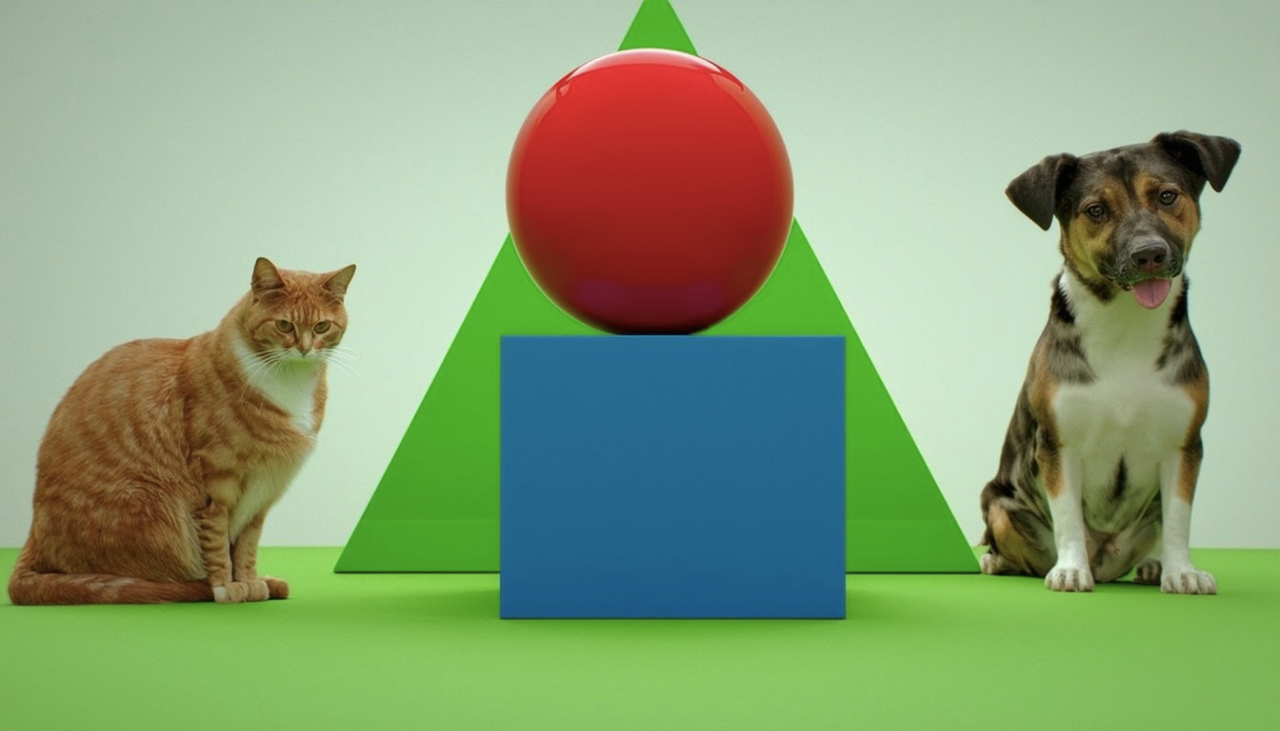
The performance of Stable Diffusion 3 thus far has demonstrated a remarkable resemblance to the prompts provided. The horse's illustration, too, even depicts the horse stepping upon the ball, the sphere undergoing deformation as a result.
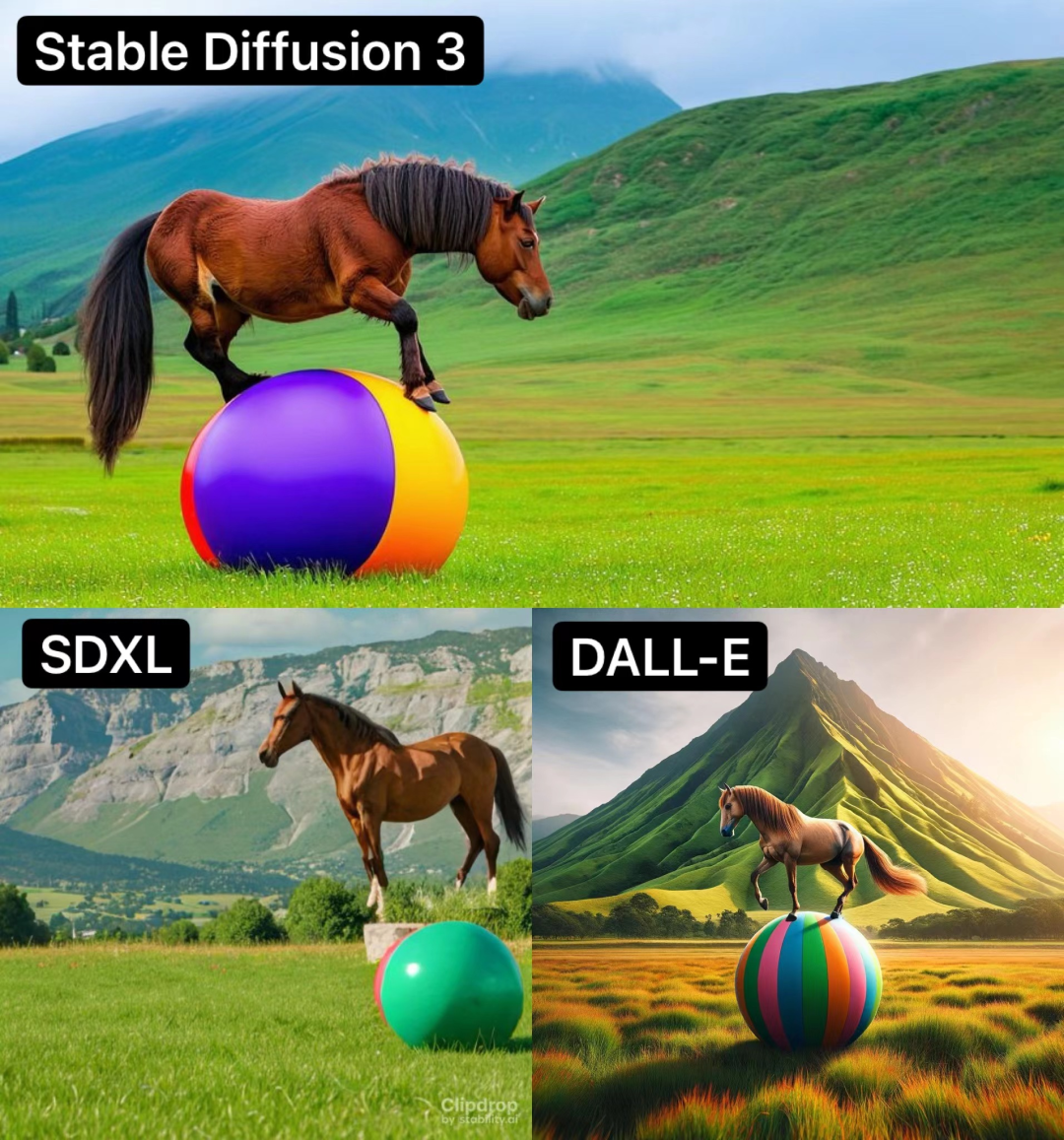
The following prompt proved a major challenge for many competitors:
Prompt: a painting of an astronaut riding a pig wearing a tutu holding a pink umbrella, on the ground next to the pig is a robin bird wearing a top hat, in the corner are the words "stable diffusion"
The prompt's myriad elements of detail, spanning the subject's attributes, and positions, and accurately depicting the minute texts, made it an arduous task. Despite the abundance of demands, SD3.0 thoroughly comprehended and successfully completed them all.
The astronaut, the pink umbrella, the pig clad in a ballet mini-dress, the wise owl with the top hat, and the text in the corner, all fully adhere to the prompt requirements. This exemplifies that the model grasps the prompt well.

Even further, a dynamic subject element can be altered without affecting the other elements:
Ultra-High Quality
On image quality, SD 3.0 also obtained a remarkable improvement.
"A photographic close-up portrait of a chromatically-changing dragon in a black-colored environment," its generated image looks something like this.
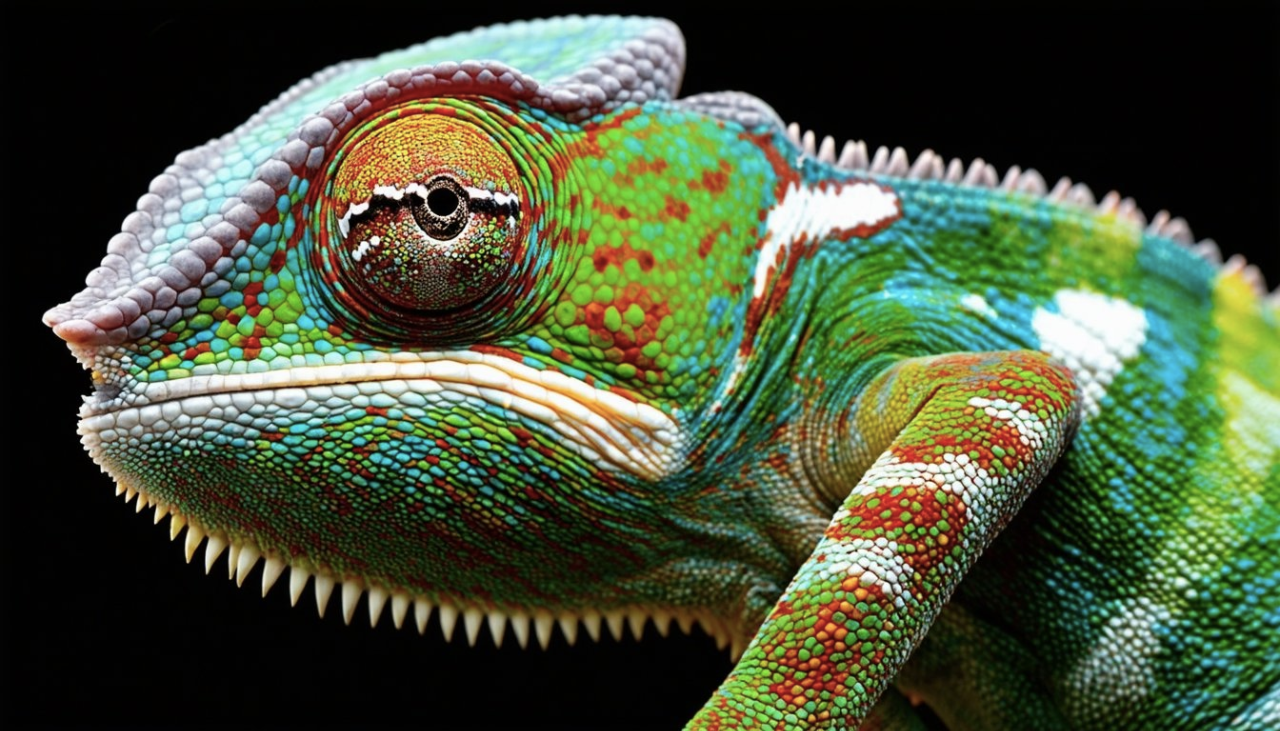
Moreover, the images generated in cartoon and sketch styles, demonstrate a stark improvement from previous versions:
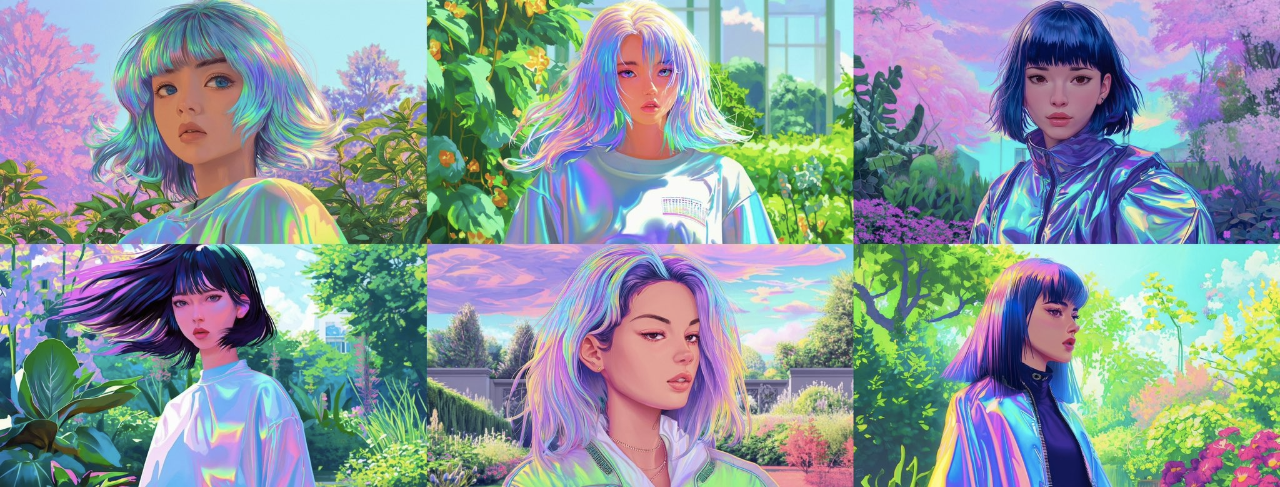
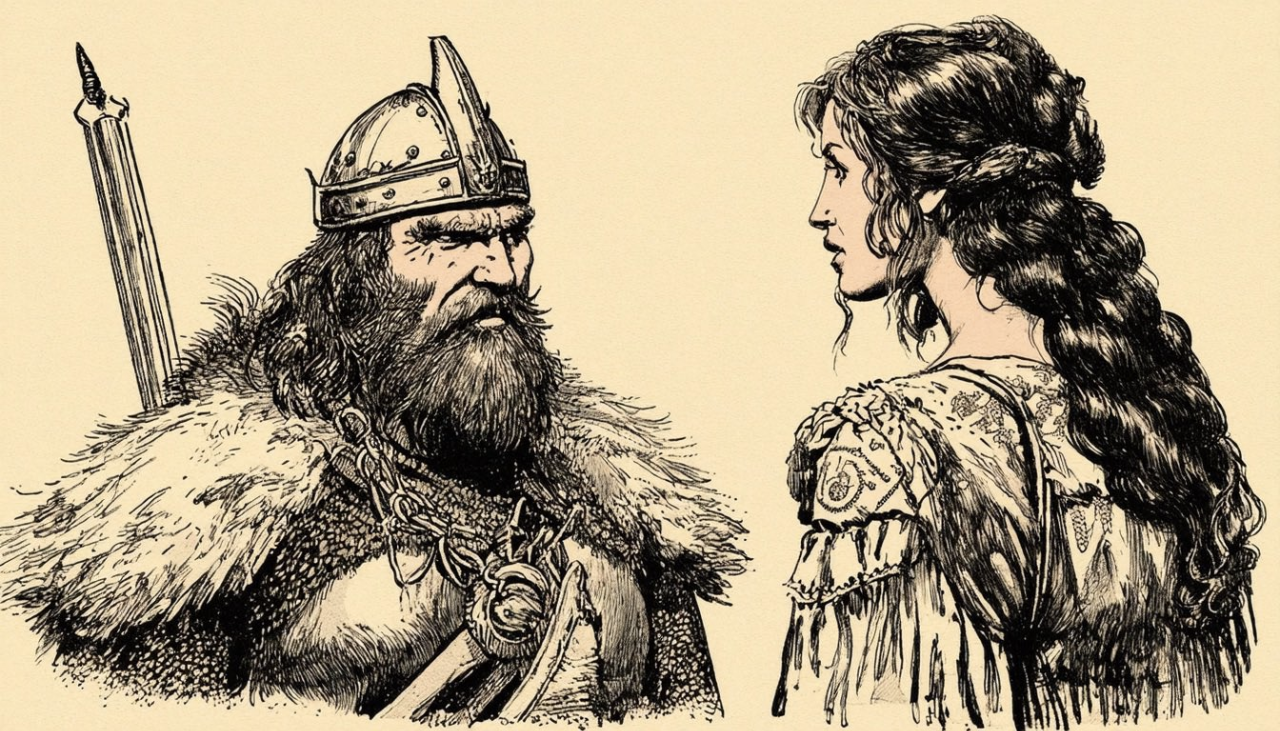
Speed and deployment
You will be able to operate the largest SD3 locally, provided that your video card possesses 24 GB of RAM capacity. The requisite threshold is likely to reduce by the time it is released to the public, and individuals will begin pursuing numerous optimization efforts on personal computers.
The first benchmark indicates 34 seconds of processing time for a 1024x1024 image with the RTX 4090 video card (50 steps). Further optimizations are feasible and will be undertaken.
New changes in Stable Diffusion 3
Noise Predictor
One notable modification of Stable Diffusion 3 contrasts its predecessors, particularly Stable Diffusion 1 and 2. Instead of the U-Net architecture used in Stable Diffusion 1 and 2 as a noise predictor, Stable Diffusion 3 employs a repetitive stack of Diffusion Transformers.
The benefit is akin to utilizing transformers in expansive language models: You can anticipate enhanced performance when enlarging the model size.
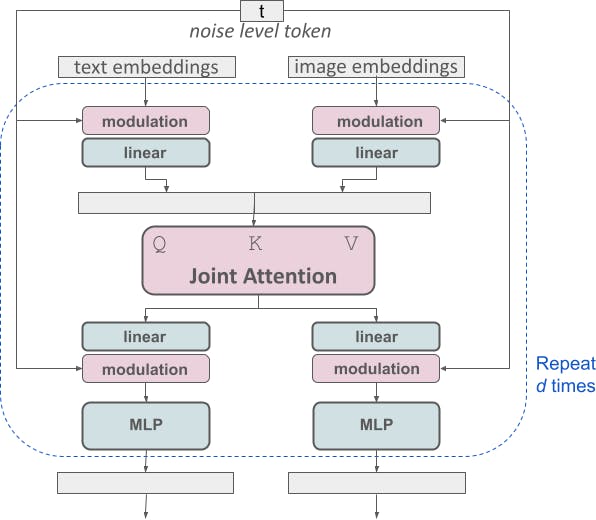
The structure of the block possesses a particular form that establishes a comparative standing between the text prompt and the latent image. It seems that this structure effectively sets the groundwork for employing multimodal variables, like visual prompts.
Sampling
The Stability team has expended substantial endeavors regarding sampling to make it prompt and effective. Stable Diffusion 3 adopts "Retified Flow sampling". Simply put, it represents the swiftest path from noise to vivid imagery — the most proficient means to accomplish this function.
The team even discovered a noise schedule that focused substantially on sampling the intermediate region of the journey, which generated finer images.
Indeed, it appears that the sampling mechanisms shall be fundamentally different. Nonetheless, probably, some extant samplers are also adequate.
Text encoders
Stable Diffusion 3 uses 3 encoders:
- OpenAI’s CLIP L/14
- OpenCLIP bigG/14
- T5-v1.1-XXL
The final element is rather considerable and may get omitted if you are not generating text content.
Better captions
One significant point is how DALLE 3 used highly precise image captions during training, which allowed it to adhere to the prompt accurately.
Likewise, Stable Diffusion 3 also undertakes this technique. As a consequence, superior prompt-followership, similar to that of DALLE 3, can be anticipated.